Finding a good protein drug candidate is often like finding a needle in a haystack. Researchers generally screen through thousands of proteins found in nature until they find one that comes close to doing what they want it to do. Then comes the painstaking process of optimizing that target so it does exactly what they want it to in the body without any adverse effects. All this is hard, takes lots of time and resources, and more than half the time fails to deliver a viable candidate.
What if scientists could optimize the process of designing better protein drugs faster and with greater success? Or better still, what if they could skip the process of finding a protein in nature and just design one from scratch, or de novo, to work the way they want it to? This is the promise that generative biology holds.
Generative biology starts by identifying the underlying rules that govern the way biological systems work. In the case of proteins, this means understanding their structure or the way that they fold in 3D. This is important because the way a protein folds determines its biological function. Researchers then use this protein structure and function data that they've collected over time to train computer models that use artificial intelligence (AI) and machine learning to make proteins with specific structures and functions. Building on this data, the computer models can eventually learn how to make new proteins that work the way drug developers want them to. The more data that is put into these models, the better, faster and more successful these models become at making desired proteins.
"We're moving from a search and discover mode of operation to a design and generate way of thinking about biological function and protein function," said Marissa Mock, director of the Generative Biology group in Research and Development.
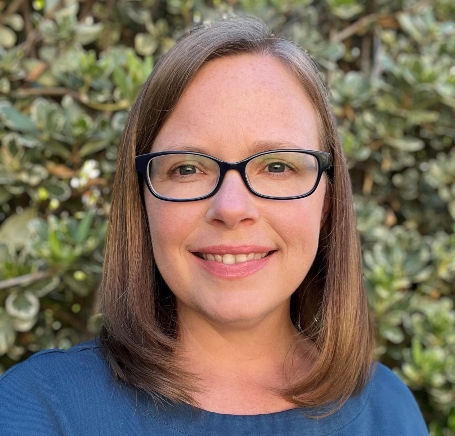
Marissa Mock, director of the Generative Biology group in Research and Development.
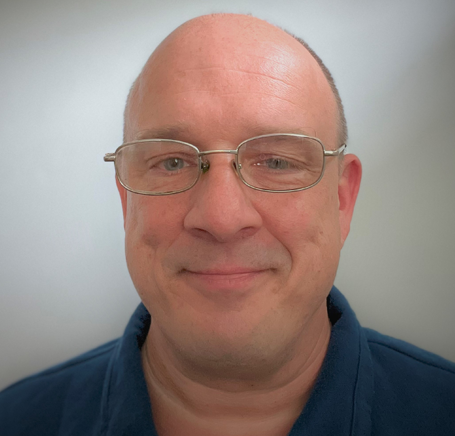
Chris Langmead, director of Digital Biologics Discovery in Research and Development.
The term generative biology stems from generative computer models. These models take data, learn from it and generate new data—in this case new protein designs. Generative models have only recently become advanced enough to apply to the field of protein engineering. "They're good enough to start to use," said Chris Langmead, director of Digital Biologics Discovery, "but we need them to get better." He added that this will not happen overnight. "It's a process. Just like human beings get better over time, so will our models."
Much like finding a needle in a haystack, researchers have traditionally used high throughput screening systems in the lab to find proteins that have the potential to become drugs. Generative computer models can allow scientists to design needles, or specific proteins, that can be tested in the lab without even needing haystacks. The more data that goes into the models, the better the output design becomes and the fewer needles the models will need to generate.
The protein folding problem
Proteins are the building blocks of life. The human body is made up of billions of them and they are essential for every basic function – from digesting food, to moving and thinking. With 20 basic amino acid building blocks, proteins can adopt all sorts of different shapes and sizes.
Proteins come in all different shapes and sizes with wide ranging functions. This great variety provides an opportunity to design and develop many different kinds of protein-based drugs. Shown are some examples of naturally occurring proteins both within and outside of a cell. Credit: The Protein Data Bank (PDB101.rcsb.org).(Click on image for interactive view.)
For over fifty years, scientists have been working on cracking what's called the "protein folding problem." Thanks to the Human Genome Project researchers can figure out the sequence of a protein – or the order of its amino acid building blocks – based on the DNA sequence used to make that protein. But they've struggled with predicting how those long chains of amino acids fold up and carry out that protein's function in the body. This is because there are so many ways a protein can fold, so it takes massive amounts of computing power to calculate that final structure.
Traditionally most labs have determined a protein's structure using imaging techniques like x-ray crystallography, that scatter x-rays off crystallized proteins. A newer, quicker and more effective technique is cryo-electron microscopy, which quickly freezes the protein so it can be viewed with an electron microscope. But this faster technique is still no match for taking a protein's sequence and having computer models predict its structure.
On July 22, 2021, DeepMind, a subsidiary of Google's Alphabet Inc., published research on its machine learning computational approach that predicted the structures of about 100,000 proteins. The AI and machine learning system, called AlphaFold, uses large amounts of protein data to learn from so it can predict protein structures based on a protein's amino acid sequence. And while the model's predictions aren't perfect, they are getting better every day.
Within weeks of the AlphaFold publication coming out, researchers at Amgen began testing the tool and applying it to predict protein structures and identify problem areas that the group had been struggling with for years.
This LCB1 protein is shown folding into its final 3D shape. LCB1 is a computer-designed antiviral protein invented at the Institute for Protein Design. Once folded, the protein binds to the spike protein on the surface of the SARS-CoV-2 virus. It blocks the virus' ability to infect cells. Credit: Ian Haydon, Institute for Protein Design, University of Washington.
RoseTTAFold is a tool similar to AlphaFold that was developed by the Institute for Protein Design (IPD) at the University of Washington. Amgen has an ongoing collaboration with David Baker, the director of IPD, to learn about designing proteins de novo. Amgen is also partnering with external protein design companies specializing in AI, like Generate Biomedicines.
While RoseTTAFold and AlphaFold were first developed to predict protein structures, these and other tools can now also be used to model protein-protein complexes. Being able to see how proteins bind to each other is a key aspect of drug development. It's one thing to figure out how a protein folds, it's another to figure out how a protein binds a target and carries out a function.
The protein data AlphaFold and RoseTTAFold use comes from the open-source Protein Data Bank (PDB), that contains experimental 3D structure data for large biomolecules such as proteins, DNA and RNA. The proteins in the PDB come from nature. And while this data is valuable, natural proteins are a long way from a viable drug candidate. That's where the experimental wet lab comes in.
Wet lab meets dry lab
Amgen has a long history of expertise in protein engineering in the lab, from the first biotech drug the company made to today's increasingly complex biologics. Over the last decade, the company has made large investments in wet lab automation and other protein engineering technologies. Groups across the company have also collected vast amounts of data on the structures and functions of proteins that can act as drugs.
"At Amgen we know what drug-like proteins look like. We know how to make them," said Mock. "We have large amounts of legacy data and the ability to generate large amounts of targeted data to deliver not just a protein that works, but a protein that works and is a potential therapeutic for a patient."
By taking this treasure trove of wet lab protein drug data and feeding it into the generative computer models of the computational "dry lab," the goal is to have the models generate a plausible drug candidate that can then go back to the wet lab to be tested. The results of those tests can then be fed back into the computer models to improve on the predictions.
One application of this process is in the development of multispecific drugs that are engineered to bind multiple targets at once. Part of the multispecific design process involves using smaller, simpler protein antibodies that can be modified to bind these multiple targets. In 2021 Amgen acquired Teneobio, which produces smaller, simpler, single-chain antibodies ideally suited for this purpose. Machine learning models can help speed this process even further.
"For a decade, we've built a protein engineering foundation of exquisite biology, analytics and automation. And we built that foundation with the knowledge that eventually, it would be turbocharged by new science, enabling us to launch a generative loop," said Alan Russell, vice president of Biologic Therapeutic Discovery.
This generative loop can save enormous amounts of time. It is already cutting antibody discovery timelines in half and doubling the success rate of the engineered proteins emerging from that loop, said Russell. By using computers to generate specific proteins predicted to bind a target, rather than making them from scratch in the wet lab, the dry lab can also minimize the experimental work that goes into finding candidate proteins in the first place.
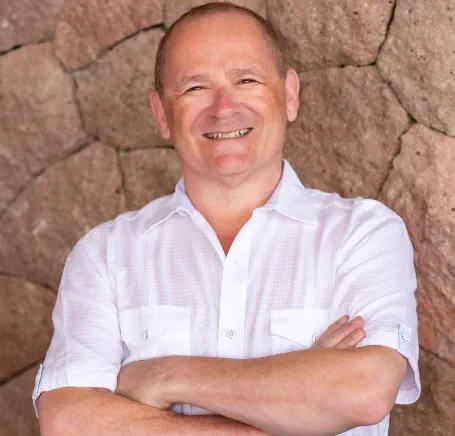
Alan Russell, vice president of Biologic Therapeutic Discovery in Research and Development.
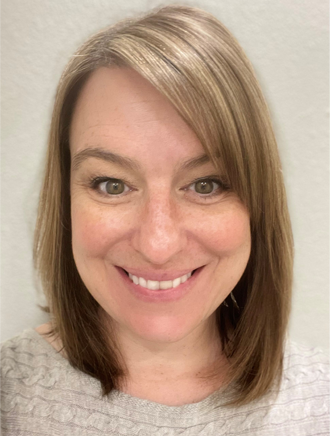
Suzanne Edavettal, executive director of Protein Engineering in Research and Development.
"All that experimental work takes a very long time. It takes a lot of resources. And if we can avoid all of that, we can go much faster," said Suzanne Edavettal, executive director of Protein Engineering. Even then, a candidate drug could still end up with undesirable effects once tested in the clinic, she said.
One example is immunogenicity, or the body's immune response to the drug. The body is exceptionally good at detecting and destroying foreign compounds, but researchers don't completely understand this complex process. During drug development, immunogenicity often can't be tested until the clinical trial phase, when a lot of effort and resources have already gone into designing the drug candidate.
The hope is that machine learning can help figure out what makes a desirable protein in fewer iterations and minimize surprises that could jeopardize the drug along the way. Decreasing cycle time and increasing probability of success are two of the greatest challenges in R&D. "The whole field of data science has been around for a long time, but it has just hit this inflection point, where now it is having very practical impacts on our work in drug discovery," said Edavettal.
But ultimately, what it comes down to are the patients. "Amgen was at the forefront of the biotech revolution," said Russell. "Just as that revolution transforms patients' lives, this next wave of drug development will allow us to create the molecules that we've been dreaming of so we can again transform the lives of our patients."