Summary:
- Generative biology pairs artificial intelligence (AI)/machine learning (ML) with innovations in biology and the lab to make medicines more quickly and effectively.
- Advances in predictive and generative AI are enabling researchers to design proteins that are more suitable to be made into drugs than those found in nature.
- New lab techniques such as human immune system tissue models have the potential to predict liabilities (such as unwanted immune responses) in protein drugs earlier in the drug development process.
- Federated learning, a data-sharing model that aims to protect companies’ proprietary information while still sharing important research data, can provide much-needed high-quality protein data to help AI/ML models design better proteins more quickly.
Protein drug development is long, arduous and costly. Drug developers have typically looked to proteins in nature as starting points, and then gone through the slow, painstaking process of shaping those natural proteins into safe, effective drugs.
But the advent of artificial intelligence (AI), advanced analytical techniques and new, innovative ways to do life science research is changing all of this through a process called generative biology.
Similar to how generative AI systems (like ChatGPT) allow for the generation of new data such as text or images from existing inputs, generative biology allows for the generation of new protein-based drugs that have desired structures and properties based on existing protein data inputs.
Learn more about how recent advances in computational and experimental life sciences are impacting protein drug discovery in this Trends in Pharmacological Sciences review article.
Researchers at Amgen have started using data collected in the lab about a protein’s sequence, structure and function to train machine learning (ML) algorithms to design drug candidates more quickly and with greater success than turning natural proteins into drugs. These designed protein candidates can then be evaluated using automated, high-throughput platforms in the lab, providing additional data to fine-tune the ML models in a type of generative loop.
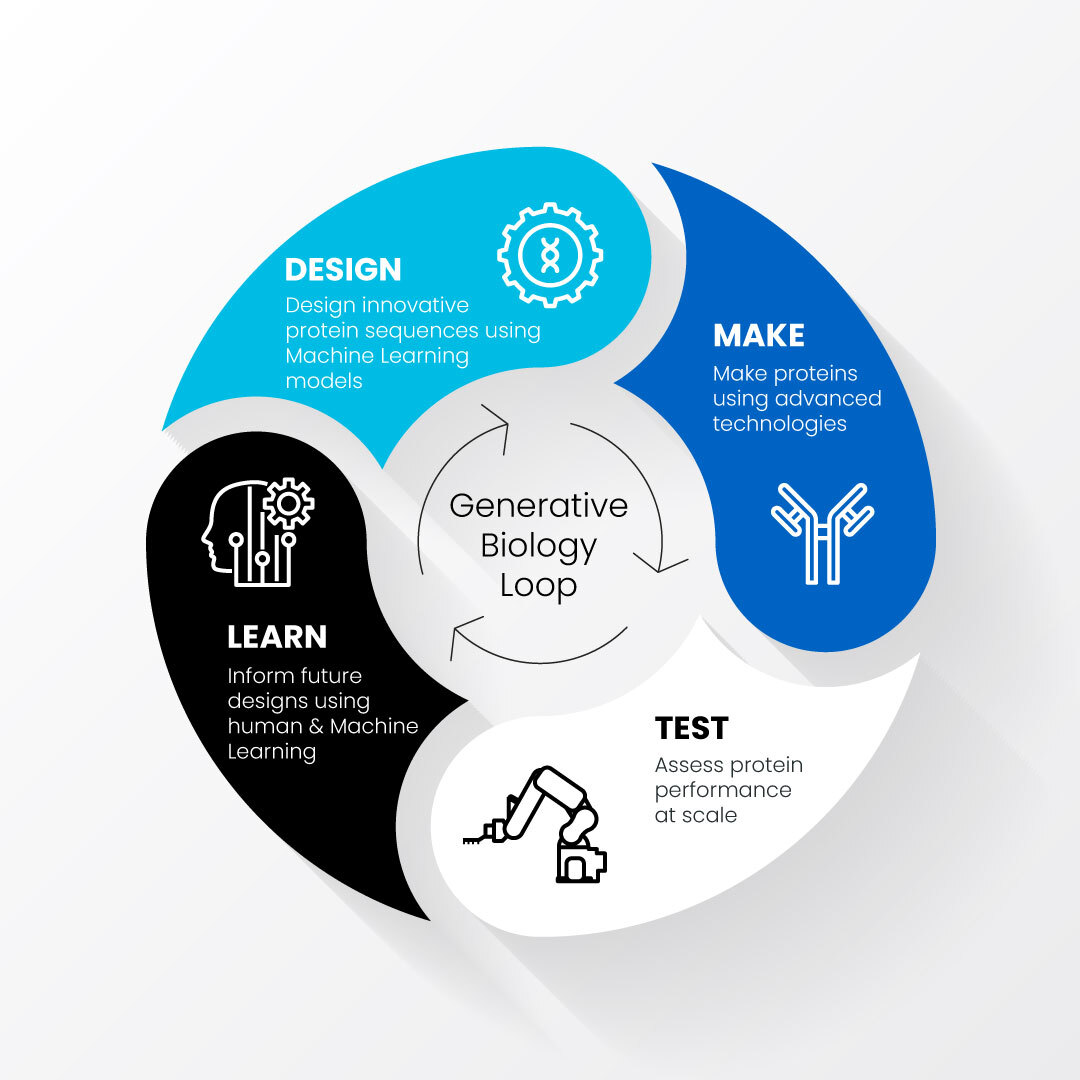
Generative biology accelerates and improves protein drug development by combining the use of machine learning-driven computational models with automated, high-throughput techniques that test protein designs in the lab. These processes of designing, making, testing and learning about proteins of interest feed into each other in a generative loop.
Improved computer modeling
Machine learning involves developing computer models that recognize and learn from patterns found in the data they are trained on. In the past, predicting patterns such as the structure of a protein was a big challenge for computer models. But over time, the models improved thanks to innovations in how to build them and as researchers began training them on hundreds of millions of naturally occurring protein sequences. This allowed the models to recognize complex patterns within protein sequences related to those proteins’ structures and functions. With advances in generative AI, some models are even capable of generating brand-new protein designs, a much-desired advance with the potential to vastly improve drug development.
To make these ML models more amenable to drug development, researchers need functional data on whether a protein binds to the target of interest. They also need data on proteins’ properties of interest to better understand their behavior. These properties may include how viscous a protein is in liquid form, how stable it is at room temperature, where it goes in the body and how the body responds to it.
From lab to patient
To determine how a protein or antibody drug will behave, researchers often make large amounts of it first. But it’s difficult and resource-intensive to make proteins in large quantities, so this part of the development process is often pushed until right before testing in the clinic (when large quantities of the protein drug are necessary to move the clinical development process forward).
Protein-based drugs are usually grown in cells that have specific sequences of DNA inserted into the cell that code for the protein of interest. To speed up this process, researchers are developing faster, more automated, and high-throughput tools that can produce proteins at larger scales and gather data from those proteins so they can be tested earlier and more quickly and thoroughly.
To predict how a protein will behave earlier in the development process and increase chances of success, researchers are also using more computational approaches. For example, Amgen has developed an ML model to predict a protein’s viscosity, an important property in drug development. If a drug is too viscous (for example, the consistency of honey), it can be too difficult to inject.
Learn more about Amgen’s protein viscosity prediction model in this MAbs publication.
To understand a protein’s properties (such as viscosity), researchers first need to consider its sequence, or the order of its amino acid building blocks. This sequence determines how a protein behaves. It has traditionally been exceedingly difficult to predict things like viscosity based on a protein’s sequence because of the complexity in predicting how such large and complex molecules will behave from their individual pieces. With the advent of generative AI and more powerful ML models, it is now possible to make those predictions.
To predict protein viscosity at Amgen, researchers used sequence data from 83 antibody proteins selected from both internal and external databases. Next, they made enough of each antibody to do extensive testing. Gathering this data, they trained ML models to use the protein sequences to predict if the antibodies had high or low viscosity with greater than 80% accuracy.
Engineering proteins that behave well under specific conditions on the shelf is important in developing a drug, but it's also important to consider how the drug behaves in a patient’s body. For example, proteins may elicit an unwanted immune response, and often the only way to tell is by monitoring clinical trial participants.
To predict human immune responses earlier in drug development, Amgen researchers are investigating the use of human tonsil organoids grown from tissue taken from people who have had their tonsils removed for medical reasons. These 3D tonsil organoids can secrete antibodies just like a human body would and could provide a better model of the immune system than the current tests that are available in the lab.
Predicting properties for proteins with a single target is already a highly complex process. But it gets even harder when trying to do it for multispecific proteins that can bind to multiple targets.
Because of their complexity, multispecific protein drugs are a challenge for ML algorithms to design and many can only be effectively made in mammalian cells, which involves a slow, rate-limiting process. They can also behave unpredictably when administered to patients. There is a lot of effort going into better ways to design and predict the behavior of multispecifics since they are an important new way to target undruggable diseases.
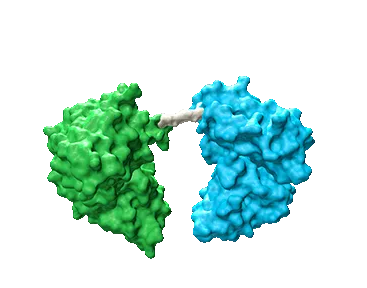
A Bispecific T-cell Engager or BiTE® molecule, is an example of a complex dual-binding multispecific medicine. BiTE molecules bind to both a T cell and a tumor cell, bringing the two together so the T cell can destroy the tumor cell. The process of designing and testing BiTE molecules is even more complicated than in protein drug candidates that have only a single target.
Wanted: More data
Training effective ML models for protein drug development requires data on many hundreds, or even thousands, of proteins. But most companies only advance an average of 3 to 12 protein drugs into clinical development per year. To obtain enough protein data for effective ML models, companies will need to collectively pool their data.
A model of data sharing, known as federated learning, can protect companies’ sensitive information, while still sharing much-needed protein data. A trusted party, such as a technology firm, could maintain a ‘global’ ML model trained on public data. That party would send the global model to each participating company, who would update it and create a new ‘local’ model. The trusted party would then combine the local models and produce an updated global model that all parties could access.
Learn more about federated learning in this Nature Comment and this JCIM publication.
By improving ML models so they produce viable designs with less, but still high-quality, data, and continuing to innovate in the lab by automating and scaling up testing, generative biology has the potential to transform the development of protein drugs and benefit patients more quickly and with greater success than ever before.